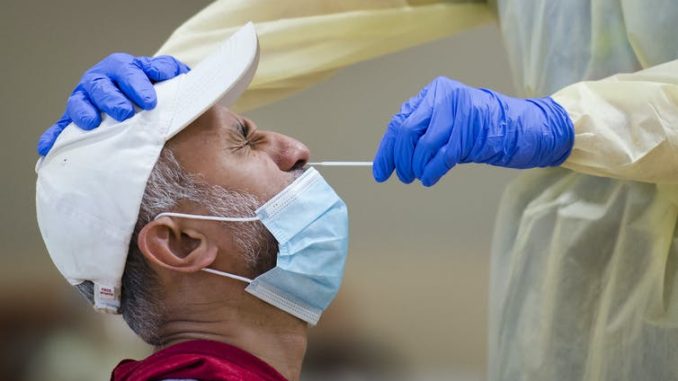
Priyanka Gogna, Queen’s University, Ontario
During the COVID-19 pandemic, words and phrases that have typically been limited to epidemiologists and public health professionals have entered the public sphere. Although we’ve rapidly accepted epidemiology-based news, the public hasn’t been given the chance to fully absorb what all these terms really mean.
As with all disease tests, a false positive result on a COVID-19 test can cause undue stress on individuals as they try to navigate their diagnosis, take days off work and isolate from family. One high-profile example was Ohio Governor Mike DeWine whose false positive result led him to cancel a meeting with President Donald Trump.
False negative test results are even more dangerous, as people may think it is safe and appropriate for them to engage in social activities. Of course, factors such as the type of test, whether the individual had symptoms before being tested and the timing of the test can also impact how well the test predicts whether someone is infected.
Sensitivity and specificity are two extremely important scientific concepts for understanding the results of COVID-19 tests.
In the epidemiological context, sensitivity is the proportion of true positives that are correctly identified. If 100 people have a disease, and the test identifies 90 of these people as having the disease, the sensitivity of the test is 90 per cent.
Specificity is the ability of a test to correctly identify those without the disease. If 100 people don’t have the disease, and the test correctly identifies 90 people as disease-free, the test has a specificity of 90 per cent.
This simple table helps outline how sensitivity and specificity are calculated when the prevalence — the percentage of the population that actually has the disease — is 25 per cent (totals in bold):
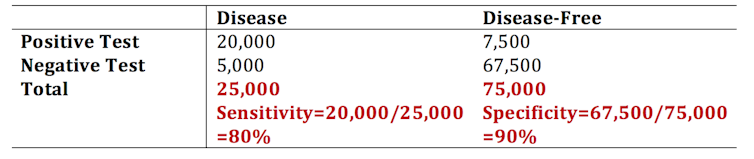
A test sensitivity of 80 per cent can seem great for a newly released test (like for the made-up case numbers I reported above).
Predictive value
But these numbers don’t convey the whole message. The usefulness of a test in a population is not determined by its sensitivity and specificity. When we use sensitivity and specificity, we are figuring out how well a test works when we already know which people do, and don’t, have the disease.
But the true value of a test in a real-world setting comes from its ability to correctly predict who is infected and who is not. This makes sense because in a real-world setting, we don’t know who truly has the disease — we rely on the test itself to tell us. We use the positive predictive value and negative predictive value of a test to summarize that test’s predictive ability.
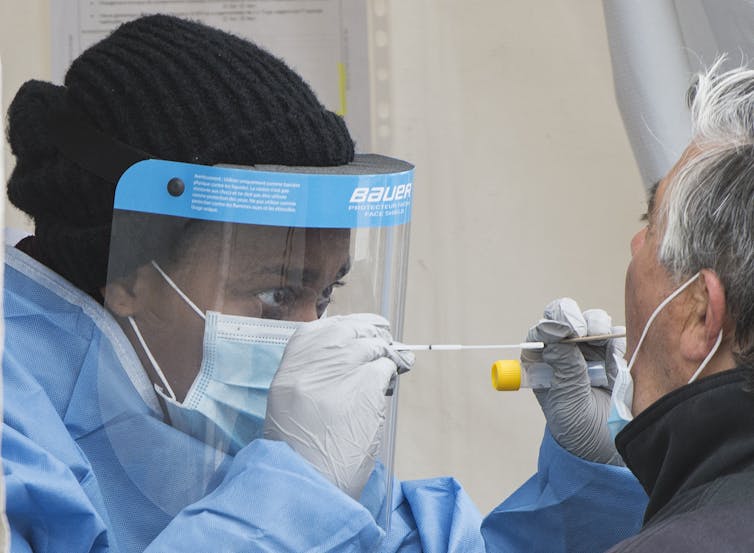
To drive the point home, think about this: in a population in which no one has the disease, even a test that is terrible at detecting anyone with the disease will appear to work great. It will “correctly” identify most people as not having the disease. This has more to do with how many people have the disease in a population (prevalence) rather than how well the test works.
Using the same numbers as above, we can estimate the positive predictive value (PPV) and negative predictive value (NPV), but this time we focus on the row totals (in bold).
The PPV is calculated as the number of true positives divided by the total number of people identified as positive by the test.

The PPV is interpreted as the probability that someone that has tested positive actually has the disease. The NPV is the probability that someone that tested negative does not have the disease. Although sensitivity and specificity do not change as the proportion of diseased individuals changes in a population, the PPV and NPV are heavily dependent on the prevalence.
Let’s see what happens when we redraw our disease table when the population prevalence sits at one per cent instead of 25 per cent (much closer to the true prevalence of COVID-19 in Canada).

So, when a disease has low prevalence, the PPV of a test can be very low. This means that the probability of a false positive test is higher. Of course, depending on the sensitivity, specificity and the prevalence in the population, the reverse can be true as well: someone that tested negative might not truly be disease-free.
False positive and false negative tests in real life
What does this mean as mass testing begins for COVID-19? At the very least it means the public should have clear information about the implications of false positives. All individuals should be aware of the possibility of a false positive or false negative test, especially as we move to a heavier reliance on testing this fall to inform our actions and decisions. As we can see using some simple tables and math above, the PPV and NPV can be limiting even in the face of a “good” test with high sensitivity and specificity.
Without adequate understanding of the science behind testing and why false positives and false negatives happen, we might drive the public to further mistrust — and even question the usefulness — of public health and testing. Knowledge is power in this pandemic.
This is an updated version of a story originally published Sept. 8, 2020. It has been updated to clarify the relationship between low PPV and false positive results.
Priyanka Gogna, PhD Candidate, Epidemiology, Queen’s University, Ontario